Beyond Static Process Descriptions
Updated: Apr 10, 2020
Have you ever used design of experiments (DoE) tools to describe your upstream bioprocess? If so you most definitely have missed the essentials of the process: the dynamics!
Here is why:
When using classic DoE tools you investigate the impact of changes in your critical process parameters (CPPs) on the critical quality attributes (CQAs). However, classic DoE tools take assumptions which are not ideal for the understanding of a process.
First, your CPPs are considered to be constant and only varied between the desired set point. Addressing, for example, the cultivation temperature inside the bioreactor, one might vary the temperature set point in a DoE between 30 and 37 degrees. However, when looking at the true process data, one can see that those temperatures are fluctuating and sometimes are out of the desired range (Figure 1). A similar situation might apply if a feeding pump of nutrients or base might, due to whatever reason, temporarily stops. While using static DoE tools, these cultivations with process variations either have to be repeated or the impact of those variations is not correctly described by the model.
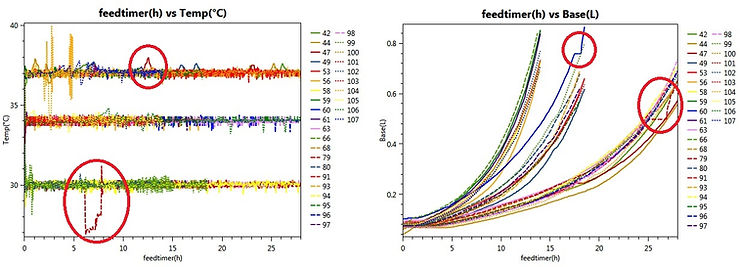
Figure 1: Temperature (left) and base feeding profile (right) of a full factorial DoE. Process variation which were included in the hybrid model but cannot be modelled using classic DoE are highlighted using red circles
Second, and more severe is the evaluation of the model with respect to the CQA. Classically, the process response is evaluated only at the endpoint (Figure 2). Hence, we assume that the input parameters remain constant throughout the process and only the process endpoint response is described by the model. Is this a clever approach? Certainly not. That’s why we are describing the process with a full process model. Instead of assuming constant input parameters and single endpoint responses, we describe the whole process using a hybrid model approach. Here, all process variations are incorporated and the whole process model is evaluated over time.

With this, it is also possible to find ideal process conditions before reaching the end of the process. Further, this hybrid modeling approach enables a faster design space description using intensified DoE (iDoE). By actively changing process parameters over time, the number of required experiments can drastically be reduced by up to 60%. As an example, we demonstrate that instead of performing seven experiments to evaluate the impact of the CPPs on CQAs (design space), four experiments can be sufficient (Figure 3).

Figure 3: Three iDoE cultivations for training and a static cultivation for model validation (left side) were used to build a model that can predict the behavior of 7 static DoE runs (right side). Here one hybrid model was generated for both the biomass concentration and the product activity (bottom).
However, the endpoint of such an iDoE cannot be interpreted in a classical way. Most of the valuable information is hidden in the response data along the process. A digital twin (i.e., an interactive model interrogation platform) can be used to simulate static process conditions and to assess the performance under static conditions. Therefore, the combination of iDoE with a hybrid modeling approach saves time and resources. The graphs displayed within this article were all generated using the pre-release version of the Novasign hybrid modeling toolbox.
If you want to test it for free, register here.